NHS AI Lab Skunkworks: the cautious early adopters
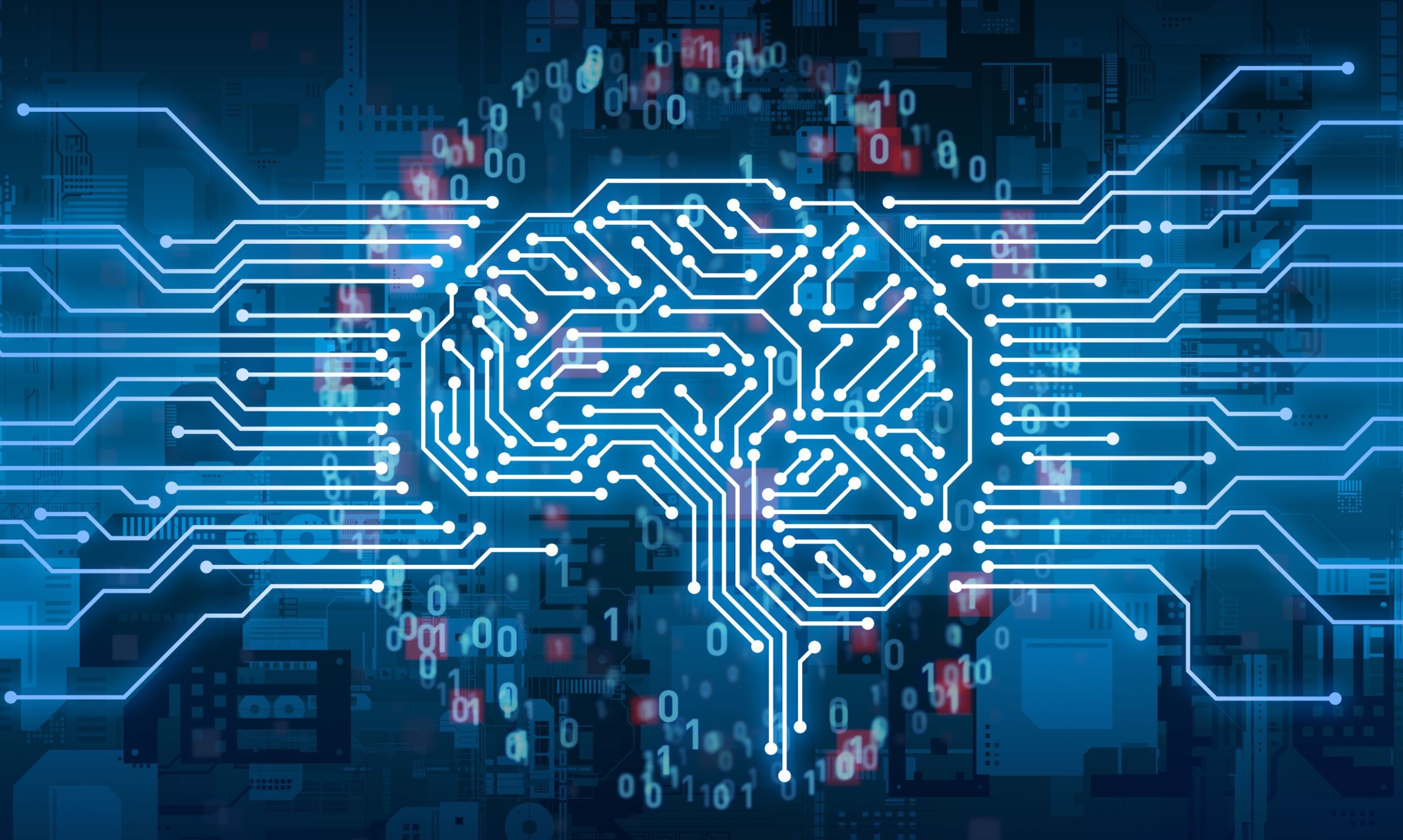
The NHS AI Lab at NHSX is keen to demonstrate the potential of artificial intelligence (AI). Hospital Times Editor David Duffy spoke to Giuseppe Sollazzo, who leads an expert NHS AI Lab team aiming to drive forward AI’s early adoption, about the need to navigate concerns, manage expectations and improve clinical workflow.
Is AI going to transform the face of healthcare overnight? Or will it inadvertently destroy it by opening healthcare data to unregulated algorithms? Probably neither.
These two questions are exaggerated demonstrations of the “cultural” barriers that often inhibit AI adoption in healthcare. “On the one hand you have certain corners of the sector that have an inherently negative view of AI, the people who think it’s almost evil,” says Giuseppe Sollazzo, Deputy Director, Head of AI Skunkworks and Deployment at NHSX, “but just as obstructive can be the group of people who consider AI to be a magic wand to addressing challenges in health delivery.”
These parallel but equally inhibiting feelings come with every technological innovation. You will have a spectrum of early adopters and enthusiasts, along with those who oppose the implementation of such innovation, due to the fear of unintended consequences.
The NHS AI Lab Skunkworks team is made up of AI and data specialists. Their brief is a fascinating one; to find new ways of applying AI solutions in both clinical and business contexts across the health and care ecosystem. Skunkworks, a small yet agile team of data scientists, works with providers to apply AI to problems and provide “proof of concept” for innovators on the frontline.
Addressing misconceptions of AI (whether inherently positive or negative in nature) is a crucial part of what Giuseppe does. He says: “Our job is to navigate these conversations, manage expectations and help ensure that the system understands AI’s relevance to them and then go about adopting it in the most sensible way.”
There are, of course, more technical barriers that arise when dealing with AI. “AI is often a challenging concept to implement, particularly that it is becoming increasingly broad in its definition and its application; these issues become amplified in healthcare.” According to Giuseppe, governance issues in both AI and healthcare frequently inhibit speedy adoption, but he insists that these are often barriers you want to have in a health system. “It helps to ensure that there are guarantees that data will not be misused,” says Giuseppe.
A self-described “open data activist”, Giuseppe previously worked as Head of Data at the Department for Transport, becoming “fascinated with improving the use of data in the public sector”. Giuseppe is no stranger to the health sector, with a decade as IT Lead at St George’s Hospital Medical School and a stint as a governor at Guy’s and St Thomas’ NHS Foundation Trust.
Case study: Recruitment shortlisting in the NHS
NHSX Skunkworks is examining the issue of bias in using AI to help compare and review job descriptions for the NHS England and NHS Improvement London Talent team.
It is hoped that AI can be applied to manage bias while improving the speed and efficiency of selection processes, leading to fairer opportunities, greater inclusivity and reductions in time and cost.
The project began with some research to explore the various existing approaches to using AI to solve this problem, from chatbots to CV screening, and automated decision-making processes to decision-making support tools, looking at the advantages and disadvantages they offer.
Source: AI Skunkworks projects
Pandemic and data sharing
Covid-19 necessitated more rapid and open approaches to data usage, with varying impacts for AI adoption in healthcare. “People understand that data is required to manage difficult situations in a much more profound way than before Covid-19,” explains Giuseppe, who points to the Data saves lives: reshaping health and social care with data draft strategy from NHSX as the embodiment of the lifesaving capability of data.
The ways in which staff can access information has been overhauled, and cultural barriers to data sharing have been swept aside in favour of the strength of clinical use cases.
Any opening of data horizons is a positive trend in favour of AI adoption in healthcare, but crisis mentality should not dictate NHS data in the long term. Central to the work of the NHS AI Lab Skunkworks is developing a normalised approach to the use of such information in healthcare. “We need to develop ‘peacetime’ data standards,” insists Giuseppe, who goes on to say that “while challenges such as Covid have helped in demonstrating the impact of cohesive data approaches, there is an increasing sense of distrust of data sharing developing – resulting in activist groups in some corners.”
Clearly the sector should not get ahead of itself by blindly following the path of innovation before trust is manifested in the public and the NHS that data is being used safely. Giuseppe affirms this: “Building trust through transparency and openness is crucial to our work.”
Community building
For those early adopters with perhaps more forward-thinking leadership in terms of AI, Giuseppe’s team ensures they are brought into the Skunkworks “community”, where insights can be more easily shared and best practice scaled in all parts of the NHS. In practical terms, this means sharing case studies and source codes for projects, joining NHS AI Lab meet-ups and contributing to regular vlog and blog posts to share insights.
This “community” has already proven to be directly beneficial to NHS AI development. The Skunkworks team is currently working on a project with the Royal Free Hospital in London and Kettering General Hospital around clinical coding. “It turned out that they have different varieties of the same issue, essentially how to automate clinical coding. Simply having these conversations as part of a community involving two hospitals with two very different sets of issues, different populations, in different parts of the country is very beneficial in showing what can be done, what can’t be done, and making sure that we develop that common language to talk about it.”
Case study: Clinical coding automation with the Royal Free and Kettering General
Skunkworks is investigating whether the process of clinical coding can be supported by artificial intelligence.
The team is providing data science capability to a joint project with the Royal Free Hospital and Kettering General Hospital. This project aims to understand which open-source models are best to support clinical coders by automating part of the clinical coding process using natural language processing (NLP) to teach computers to ‘read’ electronic health records. The aim is for the technology to summarise and suggest the standardised codes that will then be checked by clinical coders.
Source: AI Skunkworks projects
Old problems, new solutions
In the context of ominous backlog figures, NHS trusts are increasingly looking to establish how AI can help alleviate capacity concerns and free up beds. The business intelligence team at Gloucestershire Hospitals NHS Foundation Trust, supported by its chief information officer and senior clinical leaders, developed an idea to use AI to address the issue of ‘long stayers’, and applied to create a proof of concept with the AI Skunkworks.
Applying AI to issues such as bed occupancy could have profound impacts on NHS service recovery. “The fact that bed occupancy is a problem understood broadly across the NHS means that there is an abundance of good data that can inform projects,” says Giuseppe, with “business intelligence teams saying we think we can do better at predicting people who are at risk of being long stayers. And if we can predict those people and those patients, then we can target our intervention.”
The issue of bed occupancy and ‘long stayers’ falls into the category of issues Giuseppe describes as a “sweet spot”, whereby a potential solution brings immediate benefits to the patient, the hospital and to the wider system, due to the shared nature of the issue.
However, looking ahead, Giuseppe notes that the next cohort of projects that the Skunkworks team will be working on are more clinical in nature. One of these projects consists of finding ways of applying AI to predict acute kidney Injuries and prioritise observation.
As projects develop in the context of integrated care, there are interesting questions arising around what type of data should be used and how. “For each project we need to ascertain what type of data is best suited, whether it be local or national level data if we are developing more general models based on national standards, and sometimes you will need more local population-specific data. We are here to explore these questions.”
Supporting decision making, not replacing it
Being realistic about current uses for AI in the hospital sector, Giuseppe is keen to stress that in his view “currently AI in healthcare works best as a decision support tool. We are a few years away from the full trials of technologies that could be used more automatically”.
Crucial to the AI Skunkworks team is ensuring that people understand AI as a supplement to existing clinical decision making, not a replacement for it. “It’s about understanding it as an extra tool in the hands of a clinician, as radiology was an extra tool when it was first discovered over 100 years ago.”