Why you need to know how your healthcare AI works
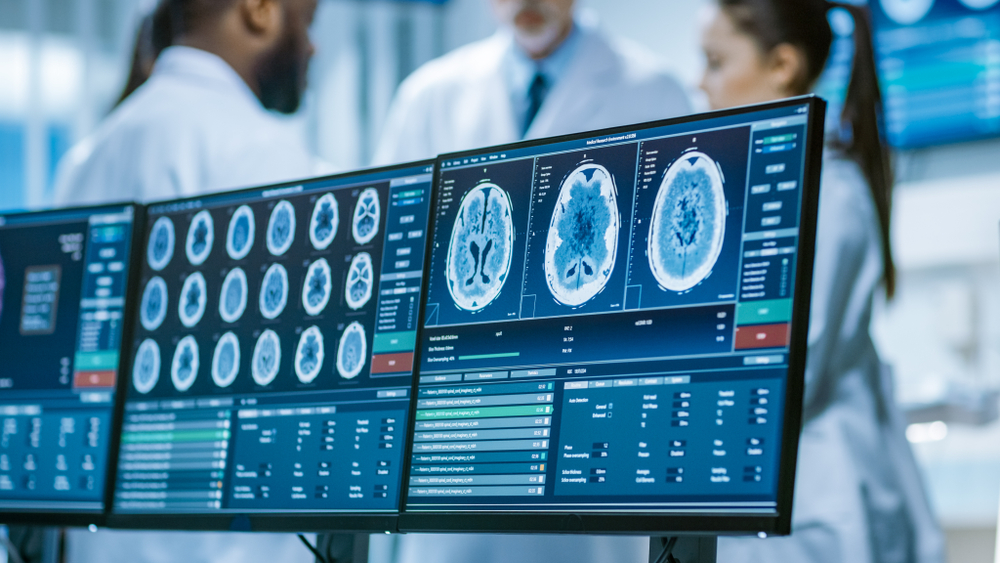
When planning to implement AI in a clinical setting to manage a specific condition, understanding how the AI will achieve its intended purpose is paramount.
Healthcare is deploying AI at scale. The NHS has published plans and frameworks for the wider adoption and implementation of AI. This holds the potential to have a hugely positive impact; AI can enhance efficiency, reduce provider workload, increase capacity, and improve patient outcomes. But for the benefits to be realised, the AI has to be designed and implemented with the right model – not just the right machine learning model, but the right operational and clinical model.
That’s where the problem lies. Many people do not understand how the AI does what it does and, therefore, what it is aiming to achieve. This makes implementing it in the right operational and clinical manner difficult.
It’s therefore important to consider some key questions and keep certain factors in mind.
What cases will the AI handle?
Taking skin cancer as an example, many will have heard something along the lines of: “If you use this AI, it can handle 50 percent of your suspected skin cancer cases, lightening your workload and boosting your efficiency!”
This may sound like a difficult offer to refuse, but is it really?
The claim is that AI will take care of half of all patients, in particular those that it thinks are unlikely to have cancer. Clinicians won’t need to see these cases again, thus reducing workload.
But which cases will it deal with? Will it deal with the challenging or tricky patient cases that may need some help diagnosing? Probably not.
More likely, it will deal with the simple stuff – the cases that are quick and easy to evaluate and probably make up less than 20 percent of the actual workload, despite accounting for 50 percent of caseloads.
While technically speaking, the technology still helps to achieve a reduction in workload, all of a sudden, the cost-benefit analysis is not quite as it seems.
If the AI cannot discharge patients, who will?
It is essential to remember that, due to current AI and healthcare regulations, a ‘machine’ is not able to make its own clinical decision. It is there to act as ‘clinical decision support’ and help clinicians in their assessment.
AI alone cannot make the decision to discharge patients, especially with conditions such as cancer.
This means that a human, i.e., a dermatologist in this example, must confirm each and every case that the AI identifies as unlikely to be skin cancer. But who decides which dermatologists provide the ‘second look’?
But now, there’s a lingering question: are hospitals paying for each patient the AI manages, or for each patient the second-look-dermatologist reviews?
While this might seem like an irrelevant nuance, it leads to a broader question: are you paying for AI technology, or are you paying for the outsourcing of your non-complex clinical cases?
It may still be of value; outsourcing models to the private sector are well embedded in the NHS and, in many cases, are win-win for all parties.
But you need to know what service you are paying for to ensure it achieves your required aims.
Is the AI truly cost-effective?
What if the AI suggests discharging a patient as they are deemed non-cancerous, but the dermatologist who is taking the ‘second look’ isn’t quite convinced?
What happens then? Does the patient end up coming back to the hospital dermatologist for assessment? And if so, is the hospital dermatologist aware that both the AI and a dermatologist have previously evaluated this patient’s lesion, with differing opinions?
Additionally, the hospital will still bear the cost of diagnosing and managing this patient.
The hospital will pay for each patient the AI manages, but some–potentially many cases–end up back in their hands, for which they will bear the financial burden of ongoing care.
Does this still reduce workload? What is the cost-benefit analysis now?
What if it makes a mistake?
Focusing on one last, and potentially expensive, question: what happens if the AI and the ‘second look’ dermatologist both suggest discharging a patient, but later the patient is diagnosed with advanced melanoma and decides to sue? Who is liable?
Presently, the liability will sit with the provider who outsourced the care to the AI technology, regardless of whether or not they’re aware of, or have viewed, the patient’s case.
Ask the right questions
As with any technology, AI can be extremely beneficial, but that should not be the standard assumption for all AI technologies.
If using AI, which will vary between different clinical conditions and their condition-specific technology, understanding how it will realise the intended benefits should be considered a critical step. How will it increase capacity? How will it enhance efficiency? How will it support patient care?
To answer these questions, it’s essential to understand not just the AI model but also the clinical and operational model within which to deploy it.
There are plans to increase the deployment of AI across healthcare, and with good reason—technology can deal with greater volumes and provide superior accuracy at significantly quicker speeds than humans.
As this deployment gathers pace, asking the right questions is more important than ever.